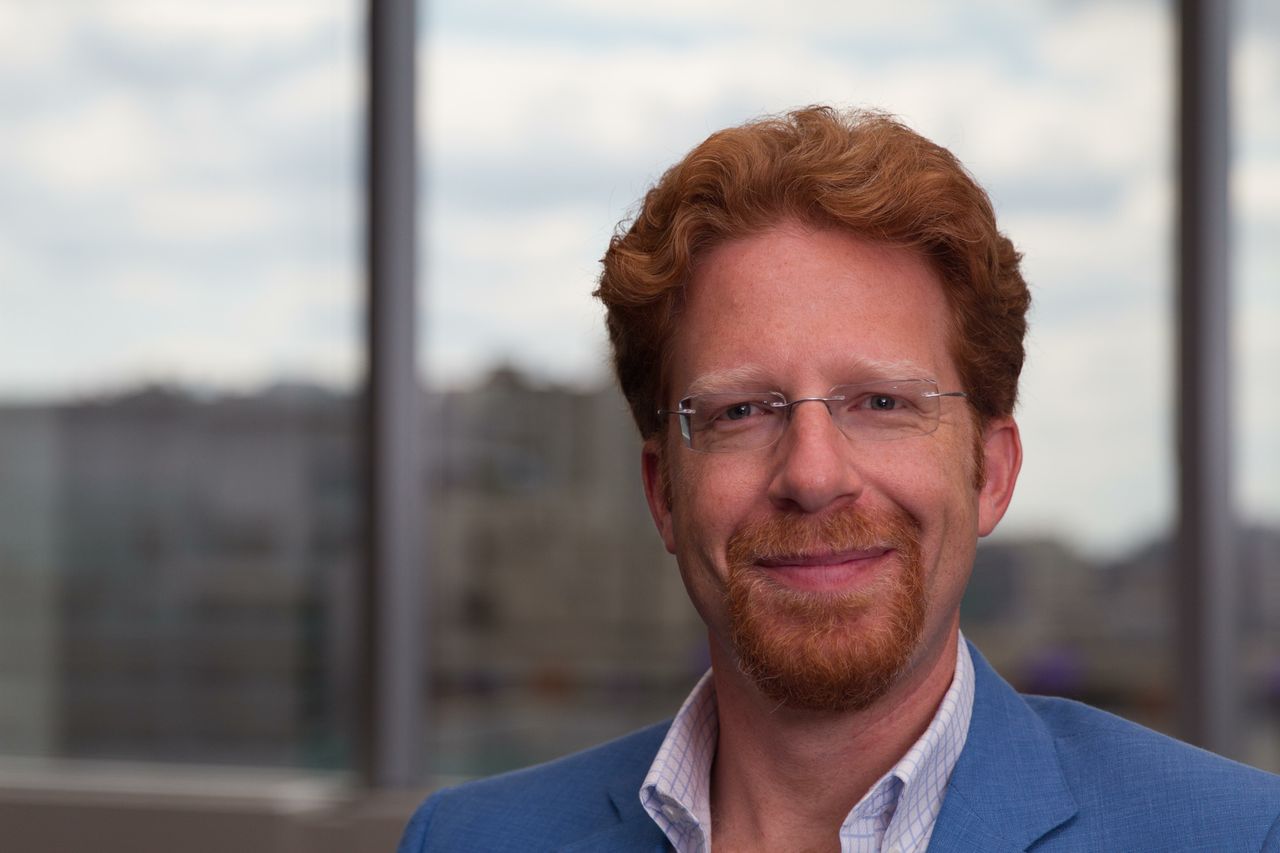
Artificial Intelligence (AI) and Simulation are two very familiar terms in the Tech world – but how do the pair partner up?
Stuart Sherman, CEO of AI company Scaled Insights and a well-known keynote speaker on topics related to AI, sat down with our CEO/CTO David McKee to discuss the differences between simulation and AI and how they can be used together for the greater good.
Can you describe the difference between Simulation and AI?
Simulation is a model whereas AI is trying to impute a model. With simulation you can build a model first and then validate it. Very often AI is trying to figure out something from nothing and simulation you build a model that you can see and test and validate and then you use that model to find information within it.
But could it be the other way round? That you use simulation to test what you think the model is to create the model?
It is similar to the Chicken or the Egg Dilemma: You could argue that building and managing the model requires assumptions to be made as well as AI for the simulation that is driving your understanding of what is happening, both of which are forms of AI. So a simulation could use AI but it can also be used by AI. It is part of the product and the solution, very much like an Ouroboros.
Could simulation be built to act as a sandbox for AI?
Absolutely. The most obvious place for that is autonomous cars where you have got the AI ready prepared. The current industry standard is to test the autonomous car by simply putting the software in, driving it on a real road and seeing what happens. By combining the existing autonomous AI with simulation you can simulate various sensors and figure out how the AI would react in circumstances with different sensors and different circumstances.
Do you think combining Simulation and AI together can help combat bias within data and decision-making?
It would be wise to put Simulation and AI together to combat bias, as it creates an infinite loop of checking where you are simulating the bias itself.
A good place to apply this would be the insurance sector as you need to find a way of running the bias against the decisions of claim adjusters. A lot of the issues of bias seem to focus on data, but why are we not more concerned around the biases within humans? Humans that are often making decisions on behalf of other people and very often only have the capacity to generalise a whole area as supposed to the individual.
By combining AI data and simulation, you can build an entire city to test an algorithm against to identify pockets that raise insurance questions and see the biases physically.
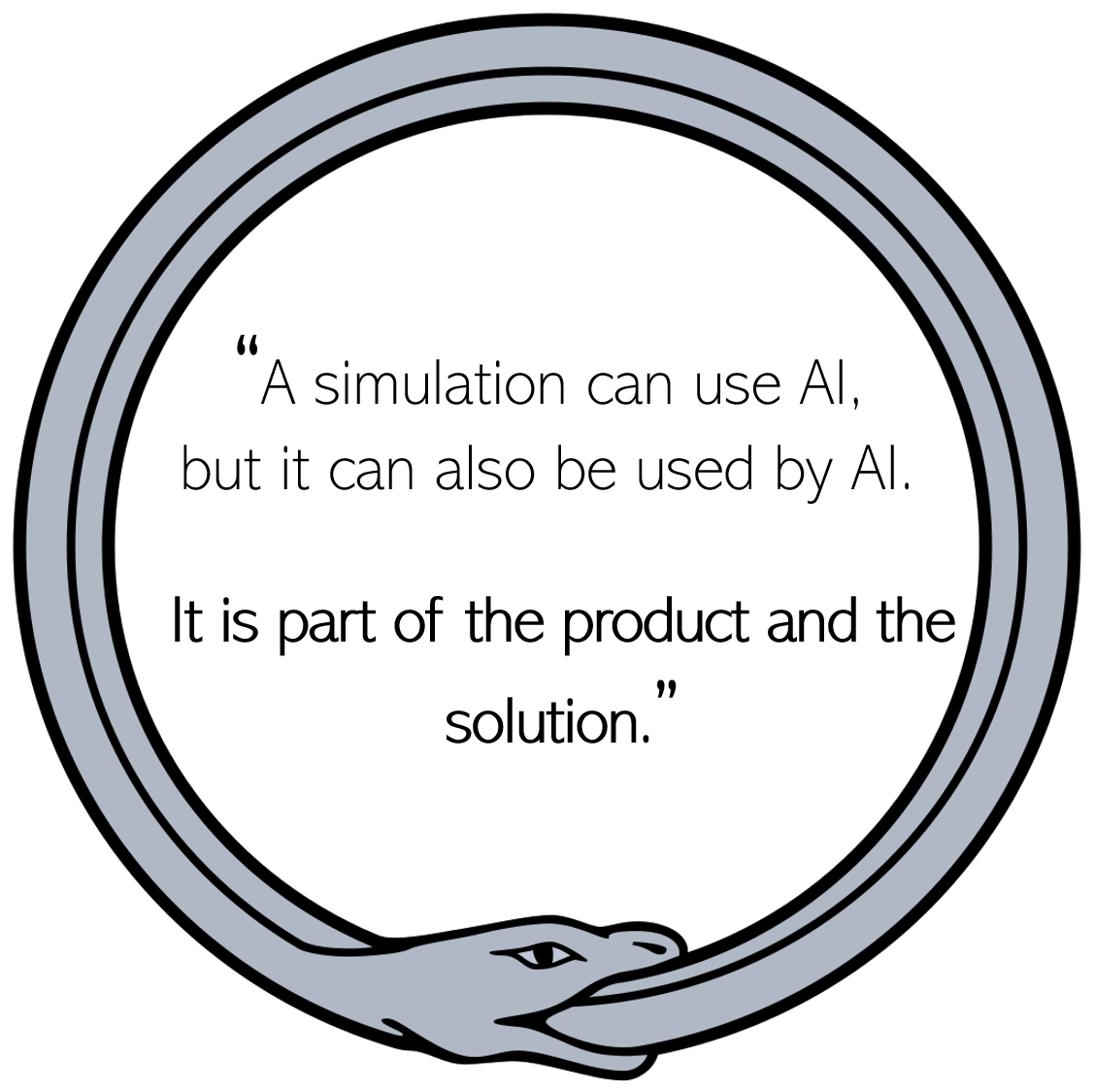
This would imply that simulation and AI need data that it often doesn’t have. Slingshot did a coronavirus simulation back in March where you see a really simple S curve however, without having that iterative process of daily updating the data, our estimations for the UK weren’t high enough because it was based off of a flat structure. If you’re trying to fit a curve, how do you find the upper bound scenario when you don’t know everything?
With something like the Coronavirus, if you’re mapping it in a simple way, you inevitably end up making assumptions. If you model it, you can start bringing in other factors generated from AI insights which then allows you to very quickly test these factors with simulation, resulting in a more multi-dimensional result.
If simulation is predicting the behaviour of a set of things over a given time period and thinking specifically about Coronavirus, what data were you using to make that simulation?
The useful thing that AI or Simulation does is that you call pull data from multiple data sources and do the correlation between them. Obviously you have to distinguish between the correlation and causation there, and then your own bias comes in to which data sources you’ve picked. So for example there’s WHO Covid-19 data in there for each country and city etc. and then you’ve also got the CIA World Factbook. Two completely different sets of data sources: CIA data isn’t time based and WHO is daily. But even then, there’s examples where that data wasn’t necessarily correct. There were elements where they had gone back and fixed the data because the reporting in each country has been different. So actually the stats aren’t fully accurate and there’s this sort of refactoring that you have to do which is really interesting of how you do that cycle of correcting the data.
And of course simulations based on these data sources don’t actually take into account the human factors which are much harder to model in terms of the decision trees for how people think.
How do you identify, and deal with, missing data?
One of my favourite examples is the “Battling Science” TED Talk where Ben Goldacre looks at ways data can be distorted within the pharmaceutical industry and what he finds is that if you plot the results of pharmaceutical studies it becomes evident that there is data missing because the pattern isn’t a normal distribution. Consequently you can see that what’s happened in pharmaceutical studies is that they’ve actually withheld studies that must have landed in these areas because they couldn’t have had studies that didn’t.
Simulation can help you fill those gaps because by rolling the simulation forward, the logical outcomes will eventually appear and you can perform a retroactive mathematical analysis to figure out what was missing. Especially if you compare the simulation to life: If I was simulating the Leeds traffic patterns you would put in all the variables then run the simulation. Theoretically if you stand on a street corner you should be able to count the cars and you should be more or less accurate with the number of cars that are passing.
But once you get to that stage, explainability becomes a lot easier as you can show that you have working model that’s exactly right which allows you to crack it open and dissect it in order to figure out the “whys”.
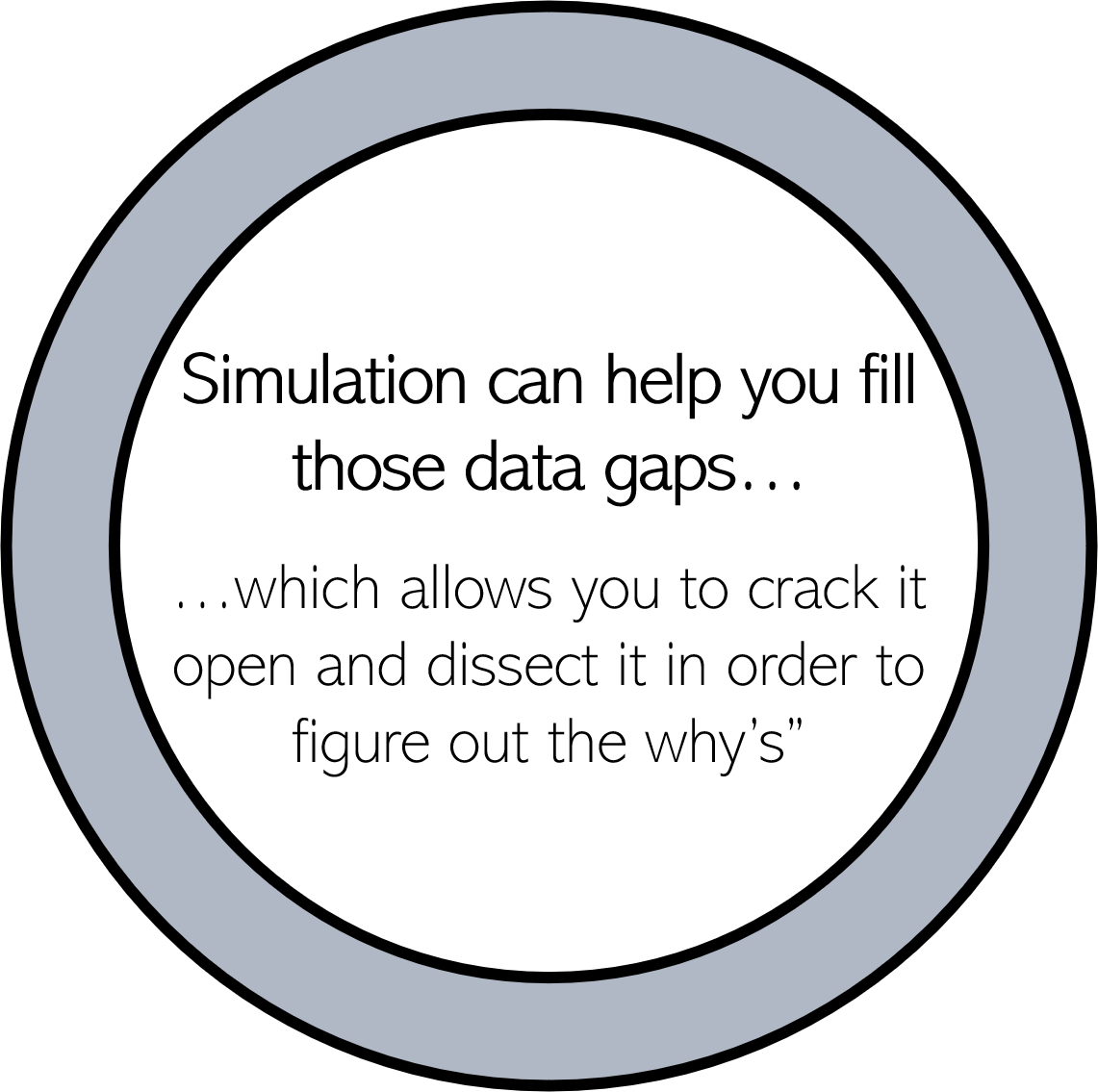
Is there a way to do behavioural modelling backwards to show all the options possible in a given scenario, and then identify the missing paths the data doesn’t show?
Providing you have enough data, yes.
A good example is the work we’re doing with Health Education England that focuses on predicting what Doctors would read. We’ve segmented Doctors into different thinking style groups and then we show them examples of things to read and we ask them the likelihood of reading one example over another, or if you could only choose one, which one would they read? From this we have been able to build a model that is about 70% predictive. So we’ve got one offering of what to read that 70% of all Doctors seem to be willing to read. If we had that kind of information a whole bunch of times we could then build that predictive model: a simulation.
But it needs to go further than this: It’s too simplistic that everything’s binary – its not just yes or no, one or the other, as there are likely to be various groups relating to certain decisions or outcomes based on genetically driven tendencies.
If we naturally have in-built biases, we should be building simulations with layers of AI insights that look at humans choices in those circumstances and predict who would make those choices, on top of the simple yes and no.
Following on from the concept of choice: One thing I noticed was that I went to step across the road in Leeds, but the people around me didn’t move therefore I stepped back. What is it that made me decide to step forward or step back?
In the context of simulation and AI is how do we capture and model these decision making processes? So how could an AI emulate the likelihood of a person stepping out onto the road?
Part of it is cultural, where for North Americans its right of way for pedestrians, and part of it is personality. Which I feel that they should stop for me. So then the question then becomes: Is there something that’s core and drives the decision and then to what degree do we put on societal niceties? And how does that play out? I love the line Dave Howlett has on this subject “Anybody driving faster than you is a maniac and anybody driving slower than you is an Ass.” So when we look at this you have your own speed and then you have a definition of people above and below so clearly you’re unwillingness to step out actually speaks of your willingness to conform and I would say that a lot of humans are very willing to conform.
How does AI see relationships within this decisions making process?
This would be an interesting thing to test in the pedestrian simulator here at the University of Leeds. Those kind of decisions that people make – there will be those kind of clusters that appear.
I think that there’s actually some interesting research into this in the behavioural economics space, specifically Dan Airely did some work at Duke University where they had a whole bunch of students come in to take a test: One of the guys was wearing a Duke shirt and he starts cheating and they look at his behaviour and then they do the study again and the guy is wearing a different university jersey. And when he’s wearing the Duke jersey other people start cheating as well. But then there was a hated school that they played football against and when the guy was wearing that hated shirt and he started cheating none of the other Duke students cheated. So it was like ‘We’re better than this guy so we don’t have to break the rules’
What I think it comes down to which is interesting is there is something deeper in the fundamental operating system of the human. So when we talk about this in terms of simulation is that these things are hard to simulate because right now we’re making assumptions about things like ‘Oh these people would cheat in these circumstances or those circumstances’ but actually what we have to do to get to the simulations you have to understand what the fundamental physics are. And if we could better define the fundamental physics of a person then we could better simulate how they would react in certain circumstances.
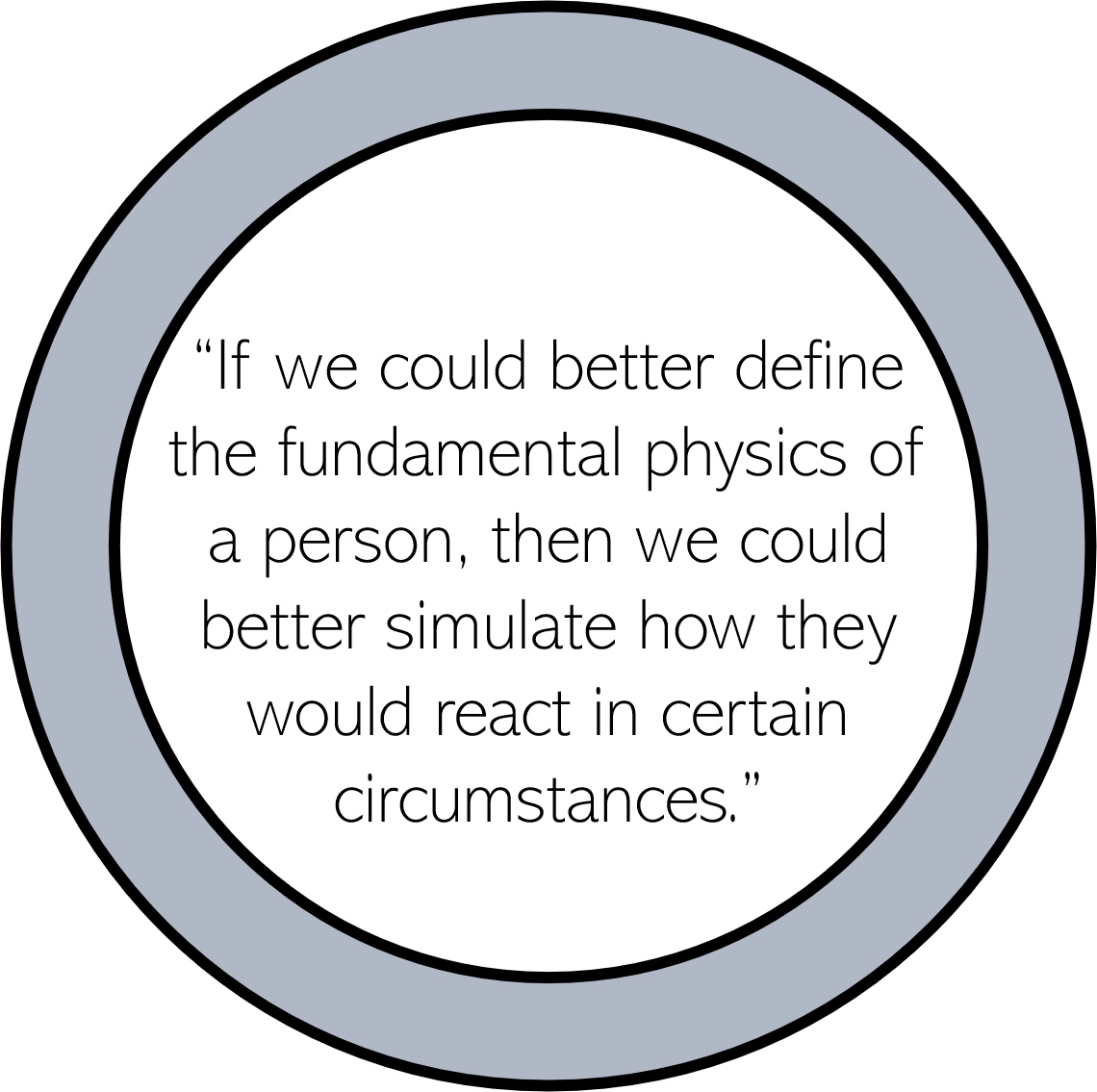
There’s constantly a question around ethics and AI. How does AI manage misinformation that has been manipulated for commercial or media purposes?
The ideal way to manage it is by gathering all available data beyond the misinterpreted and then reinterpreting it to present the viable options, which is how simulation works well alongside AI.
Using autonomous vehicles as the example again, I read a study recently on what to do with 10-year-old Tesla batteries. This is a prime example of how data has been manipulated to present a product as an environmental hero in the way of of fossil fuel reduction, whilst having a battery welded into the chassis that only has a 10 year life cycle and whose contents (Lithium Ion) are an environmental nightmare to destroy.
The best approach to misinterpreted data like that is to bring in all the possible options that don’t necessarily “look good” commercially but actually present the most risk free and well-informed option.
If we had autonomous gas powered cars that had the features of a modern car so they run a lot cleaner, efficiency of that car could improve incrementally and it would be a true hockey stick-like improvement curve would be possible and if we said ‘If these cars were hybrid we would minimise the damage by leveraging the fact that they’re autonomous to figure out how to best route plan to manage their battery packs etc.”
But data is often manipulated to turn it into a format that people can understand. Once you have presented the most risk-free option, how can you use it to sell to people in a way that isn’t promoting misinformation?
That links us to the next question: Thinking about how much you’re influencing people’s decisions: How does AI differentiate between a good idea and a bad idea?
This is where you get into the ‘Guns don’t kill people, people with guns kill people’ situation: The problem is if you have too many guns around, people get killed. Data shows. And if you don’t take care of your guns.
In this scenario what we have to do is try to make sure that our technology doesn’t get used for evil. Unfortunately the definition of evil is very broad and means something different to everyone which often means that your involvement in something “evil” can almost be inevitable, even if you’re far down the chain from the result.
By starting at the end of chain, at the result, is there a way to pre-empt a result by influencing behaviour?
I think the answer is yes because it comes down to how you inoculate people before the end result occurs. If you had a coke a week that’s perfectly fine but if you had a coke an hour that’s a problem. A good example is problem gambling, if we can identify that you’re a personality that would likely become a gambling addict that would be incredibly useful because if you knew you might make different decisions longer term especially if we introduce you to a gambling addict who said ‘look this is what it costs’, which is very different than the ‘just saying no’ American style campaigns or the British campaigns which says ‘when the fun stops, stop.’ But if you’re an addict its not about fun anymore its about a deep-seated neuro-pathway need.
There’s been a lot of traction at the moment surrounding Regulatory Frameworks and what they’re going to look like how do you think Simulation and AI can be used as part of a framework like that?
I think that if you want to build a framework you have to build a simulation first because you don’t have anything to test it against.
Netflix came up against this issue with their cover art AI. If you watched a lot of movies starring black people you were then given movies that had a 2 minute black role in it as the cover art.
It’s not that Netflix was racist, its because the AI wasn’t tested properly. When you have a team of primarily Caucasian and potentially Asian programmers testing a system out, combined with an absence of a reasonable amount of black programmers, elements like this don’t get picked up on.
It would be far better to run that in simulation so you can start sandboxing software to find the biases and find the areas that have not been considered within a Regulatory Framework. In fact, simulation and sandboxing should be almost interchangeable where you can use simulation to look at something within a sandbox context and rule issues out.